contains ()
The contains() function in PySpark is used to check if a string column contains a specific substring. It’s typically used in a filter() or select() operation to search within the contents of a column and return rows where the condition is true.
Syntax
Column.contains(substring: str)
Key Points
contains()
is case-sensitive by default. For case-insensitive matching, use it withlower()
orupper()
.- It works on string columns only. For non-string columns, you would need to cast the column to a string first.
sample DF
+-------+----------+
| Name|Department|
+-------+----------+
| James| Sales|
|Michael| Sales|
| Robert| IT|
| Maria| IT|
+-------+----------+
# Filter rows where 'Department' contains 'Sales'
filtered_df = df.filter(df["Department"].contains("Sales"))
filtered_df.show()
+-------+----------+
| Name|Department|
+-------+----------+
| James| Sales|
|Michael| Sales|
+-------+----------+
# Add a new column 'Is_Sales' based on whether 'Department' contains 'Sales'
from pyspark.sql.functions import when
df_with_flag = df.withColumn(
"Is_Sales",
when(df["Department"].contains("Sales"), "Yes").otherwise("No")
)
df_with_flag.show()
+-------+----------+--------+
| Name|Department|Is_Sales|
+-------+----------+--------+
| James| Sales| Yes|
|Michael| Sales| Yes|
| Robert| IT| No|
| Maria| IT| No|
+-------+----------+--------+
#Case-Insensitive Search
# Search for 'sales' in a case-insensitive manner
from pyspark.sql.functions import lower
df_lower_filtered = df.filter(lower(df["Department"]).contains("sales"))
df_lower_filtered.show()
+-------+----------+
| Name|Department|
+-------+----------+
| James| Sales|
|Michael| Sales|
+-------+----------+
collect ()
The collect () function simply gathers all rows from the DataFrame or RDD and returns them as a list of Row objects. It does not accept any parameters.
Syntax
DataFrame.collect()
not any parameter at all
Key Points
- Use on Small Data: Since collect() brings all the data to the driver, it should be used only on small datasets. If you try to collect a very large dataset, it can cause memory issues or crash the driver..
- For large datasets, consider using alternatives like take(n), toPandas(), show(n)
sample DF
+-------+---+
| Name|Age|
+-------+---+
| Alice| 25|
| Bob| 30|
|Charlie| 35|
+-------+---+
# Collect all rows from the DataFrame
collected_data = df.collect()
# Access all rows
print(collected_data)
[Row(Name='Alice', Age=25), Row(Name='Bob', Age=30), Row(Name='Charlie', Age=35)]
# Access a row
print(collected_data[0])
Row(Name='Alice', Age=25)
# Access a cell
print(collected_data[0][0])
Alice
# Display the collected data
for row in collected_data:
print(row)
==output==
Row(Name='Alice', Age=25)
Row(Name='Bob', Age=30)
Row(Name='Charlie', Age=35)
# Filter and collect
filtered_data = df.filter(df["Age"] > 30).collect()
# Process collected data
for row in filtered_data:
print(f"{row['Name']} is older than 30.")
==output==
Charlie is older than 30.
# Access specific columns from collected data
for row in collected_data:
print(f"Name: {row['Name']}, Age: {row.Age}")
==output==
Name: Alice, Age: 25
Name: Bob, Age: 30
Name: Charlie, Age: 35
transform ()
The transform () function in PySpark is a higher-order function introduced to apply custom transformations to columns in a DataFrame. It allows you to perform a transformation function on an existing column, returning the transformed data as a new column. It is particularly useful when working with complex data types like arrays or for applying custom logic to column values.
Syntax
df1 = df.transform(my_function)
Parameters
my_function: a python function
Key point
The transform()
method takes a function as an argument. It automatically passes the DataFrame (in this case, df
) to the function. So, you only need to pass the function name “my_function"
sample DF
+-------+---+
| Name|Age|
+-------+---+
| Alice| 25|
| Bob| 30|
|Charlie| 35|
+-------+---+
from pyspark.sql.functions import upper
#Declare my function
def addTheAge(mydf):
return mydf.withColumn('age',mydf.age + 2)
def upcaseTheName(mydf):
reture mydf.withColumn("Name", upper(mydf.Name))
#transforming: age add 2 years
df1=df.transform(addTheAge)
df1.show()
+-------+---+
| Name|Age|
+-------+---+
| Alice| 27|
| Bob| 32|
|Charlie| 37|
+-------+---+
The transform () method takes a function as an argument. It automatically passes the DataFrame (in this case, df) to the function. So, you only need to pass the function name "my_function".
#transforming to upcase
df1=df.transform(upcaseTheName)
df1.show()
+-------+---+
| Name|Age|
+-------+---+
| ALICE| 25|
| BOB| 30|
|CHARLIE| 35|
+-------+---+
#transforming to upcase and age add 2 years
df1=df.transform(addTheAge).transform(upcaseTheName)
df1.show()
+-------+---+
| Name|Age|
+-------+---+
| ALICE| 27|
| BOB| 32|
|CHARLIE| 37|
+-------+---+
udf ()
The udf (User Defined Function) allows you to create custom transformations for DataFrame columns using Python functions. You can use UDFs when PySpark’s built-in functions don’t cover your use case or when you need more complex logic.
Syntax
from pyspark.sql.functions import udf
from pyspark.sql.types import DataType
# Define a UDF function ,
# Register the function as a UDF
my_udf = udf(py_function, returnType)
Parameters
py_function
: A Python function you define to transform the data.returnType
: PySpark data type (e.g.,StringType()
,IntegerType()
,DoubleType()
, etc.) that indicates what type of data your UDF returns.
StringType()
: For returning strings.IntegerType()
: For integers.FloatType()
: For floating-point numbers.BooleanType()
: For booleans.ArrayType(DataType)
: For arrays.StructType()
: For structured data.
Key point
You need to specify the return type for the UDF, as PySpark needs to understand how to handle the results of the UDF.
sample DF
+-------+---+
| Name|Age|
+-------+---+
| Alice| 25|
| Bob| 30|
|Charlie| 35|
+-------+---+
from pyspark.sql import SparkSession
from pyspark.sql.functions import udf
from pyspark.sql.types import StringType
# Define a Python function
def to_upper(name):
return name.upper()
# Register the function as a UDF, specifying the return type as StringType
uppercase_udf = udf(to_upper, StringType())
# Apply the UDF using withColumn
df_upper = df.withColumn("Upper_Name", uppercase_udf(df["Name"]))
df_upper.show()
+-------+---+----------+
| Name|Age|Upper_Name|
+-------+---+----------+
| Alice| 25| ALICE|
| Bob| 30| BOB|
|Charlie| 35| CHARLIE|
+-------+---+----------+
# UDF Returning Boolean
from pyspark.sql.functions import udf
from pyspark.sql.types import BooleanType
# Define a Python function
def is_adult(age):
return age > 30
# Register the UDF
is_adult_udf = udf(is_adult, BooleanType())
# Apply the UDF
df_adult = df.withColumn("Is_Adult", is_adult_udf(df["Age"]))
# Show the result
df_adult.show()
+-------+---+--------+
| Name|Age|Is_Adult|
+-------+---+--------+
| Alice| 25| false|
| Bob| 30| false|
|Charlie| 35| true|
+-------+---+--------+
# UDF with Multiple Columns (Multiple Arguments)
from pyspark.sql.functions import udf
from pyspark.sql.types import StringType
# Define a Python function that concatenates two columns
def concat_name_age(name, age):
return f"{name} is {age} years old"
# Register the UDF
concat_udf = udf(concat_name_age, StringType())
# Apply the UDF to multiple columns
df_concat = df.withColumn("Description", concat_udf(df["Name"], df["Age"]))
# Show the result
df_concat.show()
+-------+---+--------------------+
| Name|Age| Description|
+-------+---+--------------------+
| Alice| 25|Alice is 25 years...|
| Bob| 30| Bob is 30 years old|
|Charlie| 35|Charlie is 35 yea...|
+-------+---+--------------------+
spark.udf.register(), Register for SQL query
in PySpark, you can use spark.udf.register() to register a User Defined Function (UDF) so that it can be used not only in DataFrame operations but also in SQL queries. This allows you to apply your custom Python functions directly within SQL statements executed against your Spark session.
Syntax
spark.udf.register(“registered_pyFun_for_sql”, original_pyFun, returnType)
parameter
- registered_pyFun_for_sql: The name of the UDF, which will be used in SQL queries.
- original_pyFun: The original Python function that contains the custom logic.
- returnType: The return type of the UDF, which must be specified (e.g., StringType(), IntegerType()).
smaple df
+-------+---+
| Name|Age|
+-------+---+
| Alice| 25|
| Bob| 30|
|Charlie| 35|
+-------+---+
from pyspark.sql import SparkSession
from pyspark.sql.types import StringType
# Register the DataFrame as a SQL temporary view
df.createOrReplaceTempView("people")
# Define a Python function
def original_myPythonFun(name):
return name.upper()
# Register the function as a UDF for SQL with the return type StringType
spark.udf.register("registered_pythonFun_for_sql"\
, original_myPythonFun\
, StringType()\
)
# Use the UDF - "registered_pythonFun_for_sql" in a SQL query
result = spark.sql(\
"SELECT Name, registered_pythonFun_for_sql(Name) AS Upper_Name \
FROM people"\
)
result.show()
+-------+----------+
| Name|Upper_Name|
+-------+----------+
| Alice| ALICE|
| Bob| BOB|
|Charlie| CHARLIE|
+-------+----------+
we can directly use SQL style with magic %sql
%sql
SELECT Name
, registered_pythonFun_for_sql(Name) AS Upper_Name
FROM people
+-------+----------+
| Name|Upper_Name|
+-------+----------+
| Alice| ALICE|
| Bob| BOB|
|Charlie| CHARLIE|
+-------+----------+
A complex python function declares, udf register, and apply example
Sample DataFrame
#Sample DataFrame
data = [ (1, 2, 3, 9, "alpha", "beta", "gamma"), (4, 5, 6, 8, "delta", "epsilon", "zeta")]
df = spark.createDataFrame(data, ["col1", "col2", "col3", "col9", "str1", "str2", "str3"])
df.show()
+----+----+----+----+-----+-------+-----+
|col1|col2|col3|col9| str1| str2| str3|
+----+----+----+----+-----+-------+-----+
| 1| 2| 3| 9|alpha| beta|gamma|
| 4| 5| 6| 8|delta|epsilon| zeta|
+----+----+----+----+-----+-------+-----+
Define the Python function to handle multiple columns and scalars
# Define the Python function to handle multiple columns and scalars
def pyFun(col1, col2, col3, col9, int1, int2, int3, str1, str2, str3):
# Example business logic
re_value1 = col1 * int1 + len(str1)
re_value2 = col2 + col9 + len(str2)
re_value3 = col3 * int3 + len(str3)
value4 = re_value1 + re_value2 + re_value3
# Return multiple values as a tuple
return re_value1, re_value2, re_value3, value4
Define the return schema for the UDF
# Define the return schema for the UDF
return_schema = StructType([
StructField("re_value1", IntegerType(), True),
StructField("re_value2", IntegerType(), True),
StructField("re_value3", IntegerType(), True),
StructField("value4", IntegerType(), True)
])
Register the UDF
# register the UDF
# for SQL use this
# spark.udf.register("pyFun_udf", pyFun, returnType=return_schema)
pyFun_udf = udf(pyFun, returnType=return_schema)
Apply the UDF to the DataFrame, using ‘lit()’ for constant values
# Apply the UDF to the DataFrame, using 'lit()' for constant values
df_with_udf = df.select(
col("col1"),
col("col2"),
col("col3"),
col("col9"),
col("str1"),
col("str2"),
col("str3"),
pyFun_udf(
col("col1"),
col("col2"),
col("col3"),
col("col9"),
lit(10), # Use lit() for the constant integer values
lit(20),
lit(30),
col("str1"),
col("str2"),
col("str3")
).alias("result")
)
# Show the result
df_with_udf.show(truncate=False)
==output==
+----+----+----+----+-----+-------+-----+------------------+
|col1|col2|col3|col9|str1 |str2 |str3 |result |
+----+----+----+----+-----+-------+-----+------------------+
|1 |2 |3 |9 |alpha|beta |gamma|{15, 15, 95, 125} |
|4 |5 |6 |8 |delta|epsilon|zeta |{45, 20, 184, 249}|
+----+----+----+----+-----+-------+-----+------------------+
Access the “result”
from pyspark.sql.functions import col
df_result_re_value3 = df_with_udf.select ('col1', 'result',col('result').re_value3.alias("re_value3"))
df_result_re_value3.show()
+----+------------------+---------+
|col1| result|re_value3|
+----+------------------+---------+
| 1| {15, 15, 95, 125}| 95|
| 4|{45, 20, 184, 249}| 184|
+----+------------------+---------+
udf register for sql using
“sample dataframe”, “declare python function”, “Define the return schema for the UDF” are the same. only register udf different.
# register the UDF
spark.udf.register("pyFun_udf", pyFun, returnType=return_schema)
# create a temporary view for SQL query
df.createOrReplaceTempView("my_table")
notebook uses magic %sql
%sql
SELECT col1, col2, col3, col9, str1, str2, str3, pyFun_udf(col1, col2, col3, col9, 10, 20, 30, str1, str2, str3) AS result
FROM my_table
==output==
col1 col2 col3 col9 str1 str2 str3 result
1 2 3 9 alpha beta gamma {"re_value1":15,"re_value2":15,"re_value3":95,"value4":125}
4 5 6 8 delta epsilon zeta {"re_value1":45,"re_value2":20,"re_value3":184,"value4":249}
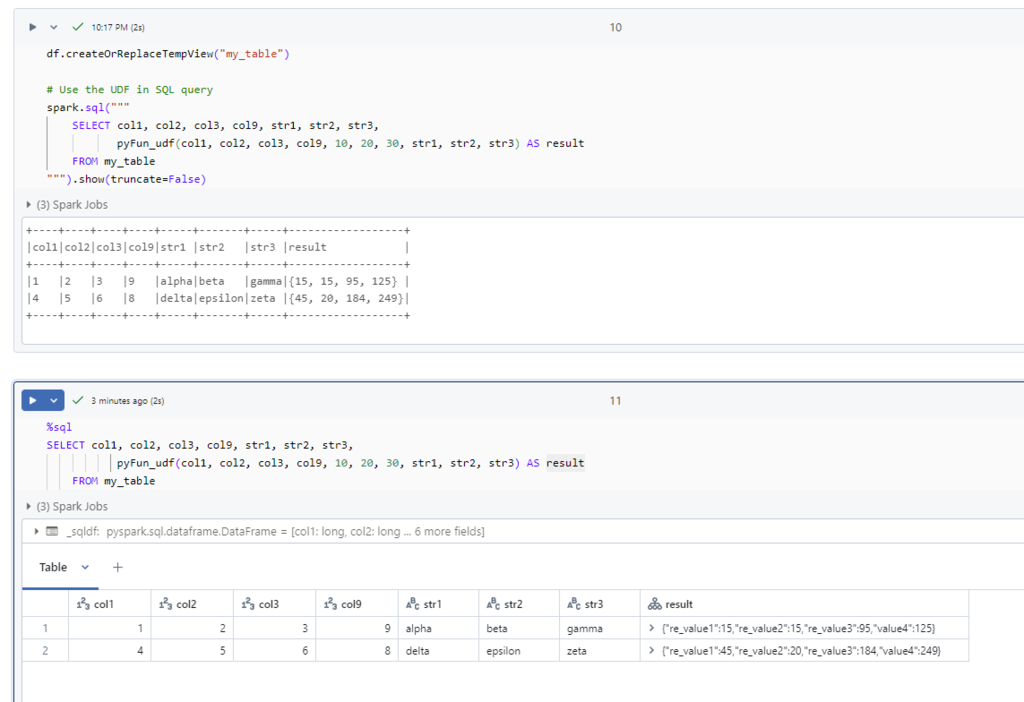